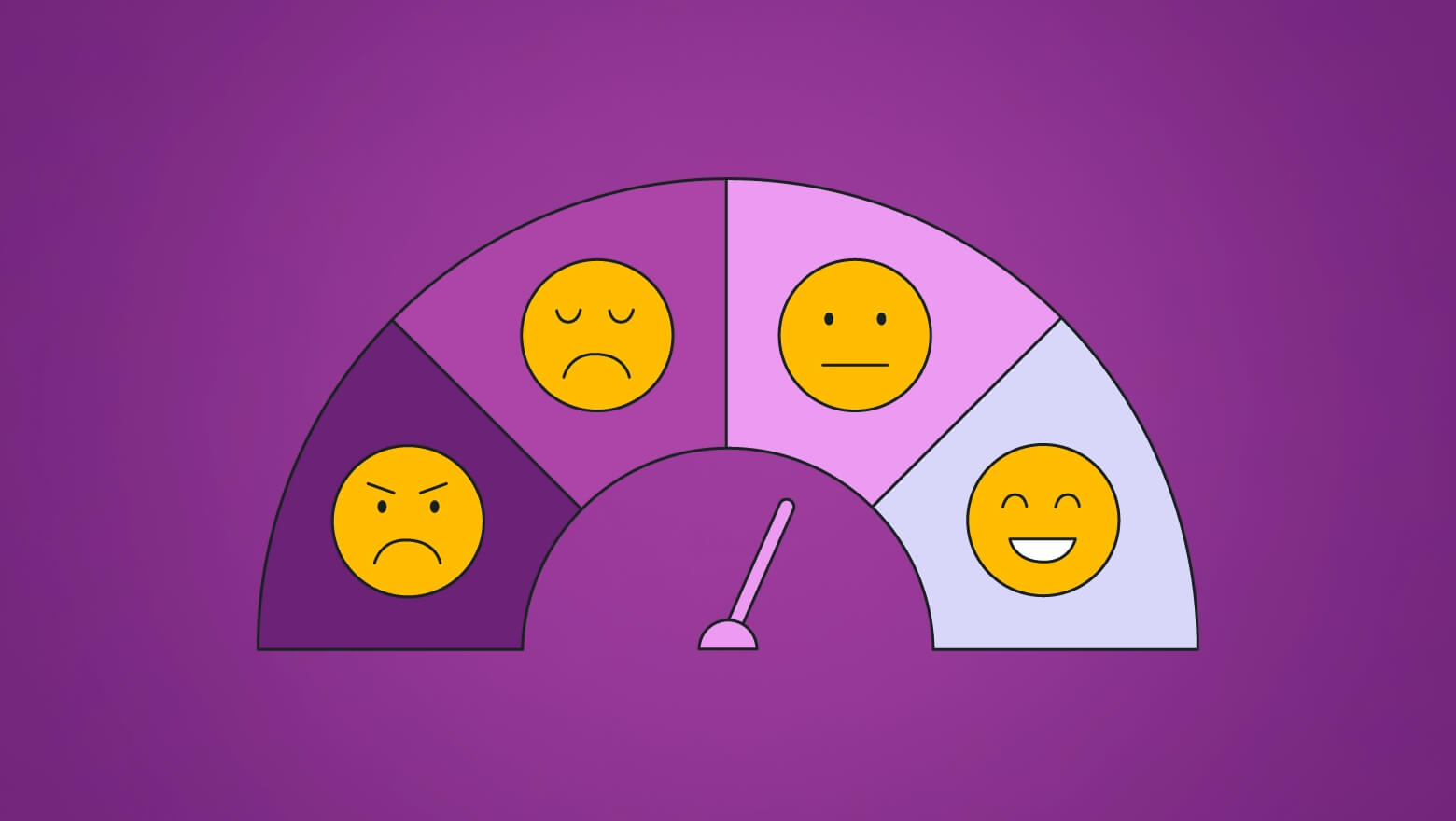
Sentiment Analysis
How to use sentiment analysis to analyze customer opinion
Reading time 9 minutes
Published on July 26, 2023
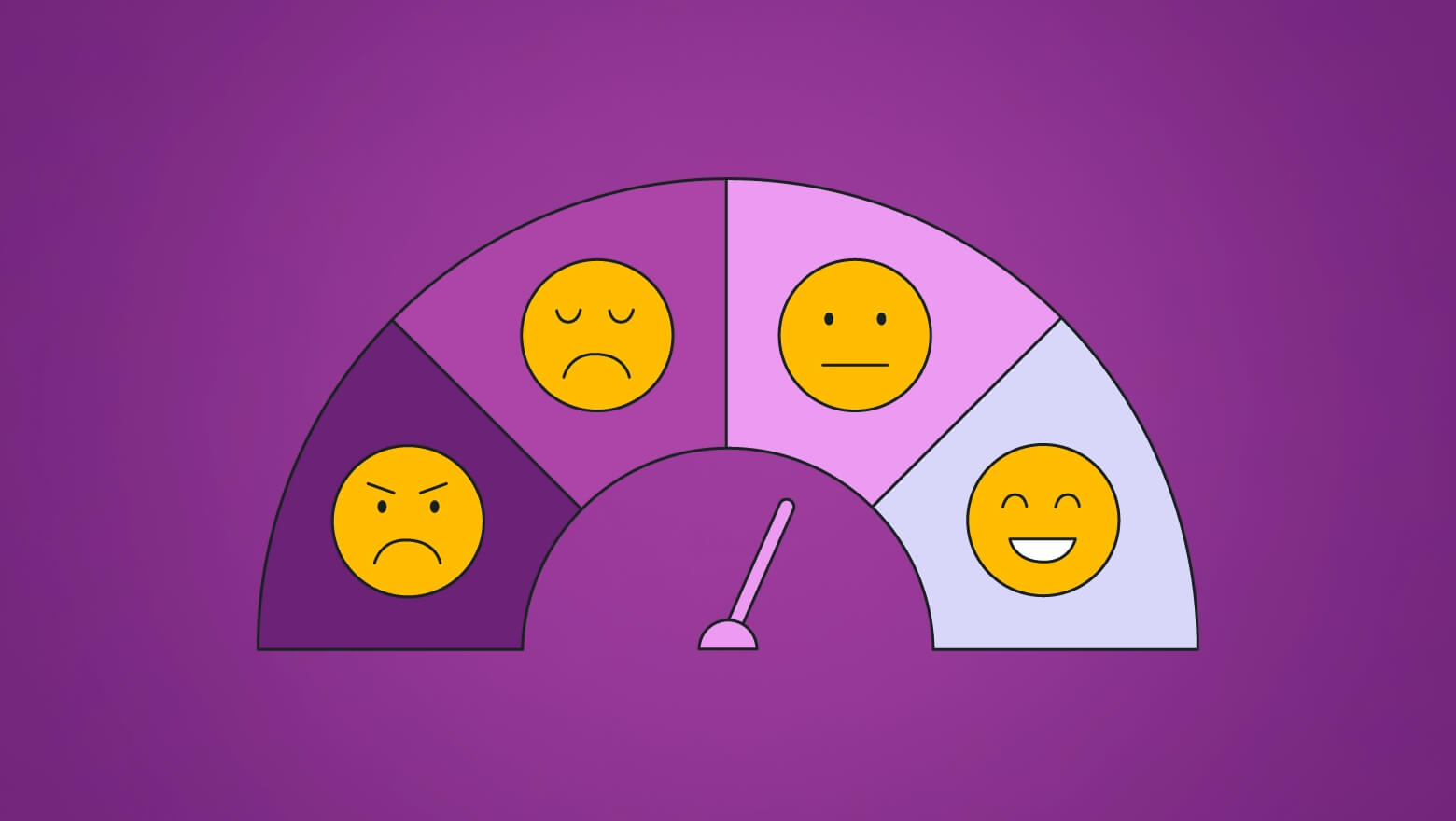
Table of Contents
The secret to a great relationship is to truly understand the other person and pay close attention to how they’re feeling. It’s the same with brands and consumers. Brands need to consistently keep a tab on market sentiment with questions like “How do customers feel about my brand?”, “Will my customers leave me for the competition?” or “What type of content do my customers like the most?”
Luckily, AI techniques like sentiment analysis take the guesswork out of the equation. In a world where customer loyalty is fickle and competition gains ground quickly, sentiment analysis enables you to efficiently discover important insights from social listening and online reviews. You’re able to find new avenues to increase market share and build meaningful relationships with your audience that drive long-lasting brand loyalty.
In this blog, we’ll take you through what sentiment analysis is, why it's critical to businesses and how to best use it to elevate your brand strategy.
What is sentiment analysis?
Sentiment analysis or opinion mining is an AI technique used to understand if the sentiment expressed in data is positive, negative or neutral. These algorithms work together with other AI tasks like named entity recognition (NER), natural language processing (NLP) and machine learning (ML) to measure sentiment in data quickly and efficiently.
Brands use sentiment analysis tools in AI marketing to surface critical information in voice of the customer (VoC) data from various sources such as social listening, reviews, surveys, contact center interactions, etc. to track brand health, improve products and enhance customer engagement and retention.
Tools such as Sprout Social have sentiment mining as a built-in feature of AI automation so you can proactively harness social insights to monitor brand health, improve efficiency and drive business impact.
What are the types of sentiment analysis?
There are three levels of sentiment analysis—document-based, topic-based and aspect-based. Let’s break them down.
Document-based sentiment analysis
This is basic opinion mining used to analyze a simple sentence and tell you whether it is negative or positive.
Topic-based sentiment analysis
Topic-level sentiment analysis is used for more complex data. It breaks down a complex sentence or paragraph to identify recurring words and phrases and classifies them into topics. It then measures sentiments for each topic separately.
When used to analyze customer comments on social media or review platforms, topic analysis gives you a better understanding of the key points mentioned in the text, which you can then track.
Aspect-based sentiment analysis
The most advanced of the three, aspect-based sentiment analysis uses semantic classification to understand comments that may not have explicitly positive words such as “love” or “hate” and tell you their sentiment.
Apart from identifying and classifying topics such as “food” or “drink,” as in the example below, it further breaks down aspects within those topics to measure sentiment. This gives you granular insights that you can use to inform business decisions.
Challenges in sentiment analysis
Sentiment analysis relies on how well a machine learning model is trained to analyze data. But even with advanced versions, certain issues can lead to inaccurate results. These include:
- Sentiment polarity: Sentiment analysis can easily identify keywords that denote emotions such as “love”, “hate” or “best”, which have high positive (+1) and negative (-1) polarity scores. The challenge arises when the text has phrases such as “not so bad”, which the model does not recognize. This results in an inaccurate sentiment score.
- Sarcasm: People use irony and sarcasm in casual conversations and memes on social media. Machine learning does not recognize back-handed compliments as negative and can misconstrue a sarcastic remark as positive.
- Emojis: Sentiment analysis depends on natural language processing and text analysis for sentiment mining, but comments on social media can be inundated with emojis. To overcome this problem, you need tools such as Sprout that will identify emojis in text data and take them into account while measuring brand or product sentiment.
- Figures of speech: Machine learning programs don’t understand figures of speech such as idioms and metaphors. For example, an idiom like “under the weather” will make no contextual sense to a sentiment analysis tool. It will disregard the text and this decreases the accuracy of results.
- Negations: A sentiment mining tool cannot recognize double negatives in sentences such as, “I can’t not have my Starbucks!”. In such cases, the tool will either disregard the comment or classify it as negative, thus skewing the sentiment score.
- Comparative sentences: Comparative sentences are tricky because they don’t really specify whether the customer is happy or unhappy unless specified. For example, the comment “The Galaxy S20 is larger than the Apple iPhone 12”, can be construed as positive or negative based on the customer’s preference.
- Multilingual sentiment analysis: When comments are in multiple languages, it compounds the challenges of a sentiment analysis algorithm. Luckily, this problem is solved when the tool has built-in native multilingual sentiment mining, like Sprout.
How is sentiment score calculated?
Sentiment scores are measured in polarities, where -1 is negative, 0 is neutral and 1 is positive. These can be calculated in three ways:
Word count method
In this method, the sentiment scores are calculated by reducing the number of negative occurrences from positive ones. For example, 1 - 2 = -1. Thus, the sentiment measured is negative. This method is used for simple texts.
Sentence length method
The score is calculated based on the length of the sentence, where the total number of positive occurrences is subtracted from negative ones and then divided by the total number of words. For example, 1-2/42 = -0.0238095. This method is usually used for complex sentences and longer comments.
Word count ratio method
This is the most popular way of calculating sentiment scores. The total number of positive occurrences in the text is divided by the total number of negative ones and added by one. For example, 1/ 2+1 = 0.33333.
Ultimately, all these separate instances of scores are calculated in a percentile form to give you the overall sentiment score of your brand, product or customer experience.
What are the sources of sentiment analysis data?
Here is a list of essential voice of the customer (VoC) data sources to use for gathering customer sentiment insights.
- Social media listening: Social listening enables you to tap into the unfiltered thoughts and opinions of your customers. This is a great way to get deeper insights into how your customers feel about your brand, content or customer service. With Sprout, easily measure this sentiment from a range of networks like Twitter, Facebook and Instagram, all in one centralized platform.
- Review platforms: Business review platforms are a key source for measuring brand health. Sprout enables you to analyze several review platforms like Google My Business, Yelp, TripAdvisor and even Glassdoor to collect actionable insights.
- News: Tap into news articles to track sentiment for your brand and competitors.
- Podcasts: Podcasts are a popular source for collecting sentiment insights on trending topics that may indicate emerging industry trends.
- Surveys: By analyzing survey responses for sentiment, you get holistic brand insights that go beyond net promoter scores (NPS) or star rating systems.
- Customer service interactions: Customer care emails, call logs and tickets are a key source of tracking and addressing the common themes in customer complaints.
- Other text sources: Sentiment analysis is possible on any data available in text. Prominent among these in various industries include electronic health reports such as healthcare data and research papers, public information as in government websites and platforms, and even gaming sites like Twitch.
Why is sentiment analysis important?
A sentiment analysis tool can help businesses leverage AI in the following ways:
Speed and scale
Sentiment analysis tools process hundreds of comments in minutes and handle millions of API calls daily. This enables brands to get timely customer insights to monitor brand performance to grow and engage audiences.
Accuracy
Machine learning algorithms calculate sentiment from vast amounts of scattered customer feedback data and measure far more scores accurately than if done manually. It also reduces the scope of biases through human emotion that can creep in. For example, a store manager who may ignore a negative comment because its from a former employee.
Topic and aspect analysis
You’re able to uncover trending topics and aspects of your business from conversations across social media networks and review platforms through hashtags and keywords and understand brand sentiment. These precise, actionable insights enable you to enhance products and offerings, customer service and other aspects of your business to improve brand performance.
You also get insights through word clouds, which give you a quick view of the important themes and topics in customer discussions around your brand. And being visually striking, word clouds are easy to understand and apt for sharing amongst stakeholders in the broader organization.
Analyze multilingual VoC data
Multilingual data is common in social listening. Sentiment analysis tools enable brands to understand culturally and ethnically diverse customers for a holistic understanding of brand sentiment.
Measure KPIs
You’re able to measure key performance indicators with precision to know what aspects of your strategy are working and which need to be improved. This is essential to measure the effectiveness of your marketing campaigns and make suitable changes in real time to ensure success. Similarly, you’re able to manage brand perception and draw brand insights by keeping track of sentiment in social mentions and customer reviews.
How does sentiment analysis work?
Sentiment analysis is a complex process so we’ve broken it down into three essential steps.
Step 1: Data collection
Choosing where your VoC data comes from is an important decision that will affect the accuracy of your insights. As a general rule, social and online platforms frequently used by your most valued customers are the best source. Once you’ve chosen the platforms you want to harness, the sentiment analysis tool natively taps into the data source either through live API integration or data scraping.
Step 2: Data processing and analysis
Once the data is collated, it undergoes data processing through machine learning to turn the unstructured information into an appropriate format for text analysis and data mining.
AI tasks like named entity recognition (NER) identify brand mentions, locations, currencies and other information relevant to the insights you want to gather. Semantic search algorithms enable the tool to understand comments contextually and avoid duplicate entries while calculating sentiment. NLP enables the tool to recognize terms that denote emotions in the text pipeline. And part of speech (PoS) taggers ensure the non-English data is natively analyzed for multilingual sentiment analysis.
Finally, sentiment analysis tasks calculate the sentiment polarity of each clustered topic and aspect and eventually give the overall sentiment score of the data.
Step 3: Data visualization
The results are now showcased in the form of charts and graphs for ease of understanding. These visualizations can be shared with other departments like marketing and customer service to improve brand engagement and address customer suggestions and pain points consciously.
Business applications of sentiment analysis
Here are some important ways in which sentiment analysis helps brands monitor emerging trends and keep ahead of competitors to build brand reputation, enhance customer loyalty and stay ahead of competitors.
Monitor industry trends
Brands have to constantly keep up with changing industry trends and customer preferences. Sentiment analysis helps you monitor social and online chatter simultaneously from multiple platforms to track emerging trends across timelines. This enables you to be ahead of the curve and proactively improve your products and services for maximum return on investment (ROI).
Conduct competitive analysis
Sentiment analysis helps you examine conversations about competing brands to track their strengths and weaknesses and inform your business strategy. You also get market-gap insights so you can explore ways to capture new business. With Sprout, see a side-by-side competitor comparison of key performance metrics across social such as share of voice, engagement, impressions and user sentiment all in one place. For even more detail, competitive monitoring is an effective way to gather and understand how your competition are outperforming your brand.
Create engaging content
Create compelling content that resonates with your customers and outperforms your competitors. Analyzing sentiment in social listening and review data for hot topics enables you to build closer relationships with your audience with personalized content based on what they’re actually talking about.
Nurture employee advocacy
Maximize brand amplification and level up employee advocacy by automating the delivery of impactful content to employees, like Medallia did using Sprout. Use sentiment analysis to know what inspires audiences the most helps you build a holistic strategy to reach a bigger audience with automated content distribution.
Improve brand reputation
Get timely alerts on spikes in brand mentions so you know when there’s an increase in online chatter about you and track the cause. Positive brand mentions like customer recommendations or praise are equally important to track as it enables you to reshare positive mentions to amplify your brand and build social proof.
Similarly, set alerts based on keywords or for a particular brand campaign to measure market sentiment and take necessary action based on your insights.
Triage inbound messages
Quickly identify incoming messages from frustrated or unhappy audience members so that customer care teams can prioritize accordingly and act swiftly. Sprout users on the Advanced Plan can tap into AI-powered sentiment in the Smart Inbox and Reviews Feed. Posts will automatically be assigned a positive, neutral, negative or unclassified value, making it seamless to isolate messages and even assign Automated Rules according to sentiment.
Gather brand insights more efficiently
Continuously monitoring and measuring customer sentiment is important to enhance your brand perception and deepen customer loyalty. It’s the most sustainable way of ensuring long-lasting customer relationships for revenue generation.
Social data helps you do this by spotting rising trends and topics amongst your target audience so you’re able to swiftly filter relevant data and tailor your campaigns for success. Analyze sentiment in keywords and hashtags across platforms like Instagram, Twitter, Reddit and YouTube to gauge customer satisfaction and level up brand performance.
Dig deeper into social listening and see how sentiment analysis helps you achieve this.
Additional resources for Sentiment Analysis
Harnessing X (Twitter) sentiment analysis for strategic business insights
Top 15 sentiment analysis tools to consider in 2024
How to analyze customer sentiment to improve customer experience
How to use sentiment analysis to analyze customer opinion